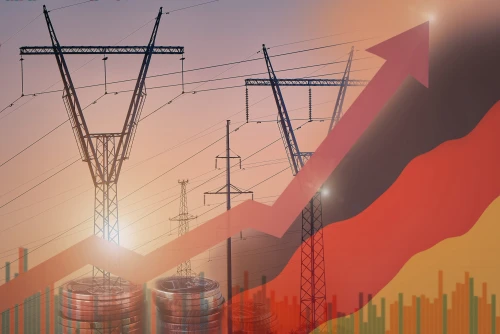
April 24th, 2025
Join our Italian Energy Day on 20 May – Book now
It might not be the first thing to come to mind when considering factors that can affect energy prices, but weather can greatly influence energy markets. From electricity generation to heating demands, the relationship between meteorological conditions and energy usage is intricate and certainly impactful. Understanding this relationship is essential for stakeholders across the energy sector, as weather conditions can drive significant fluctuations in energy prices, affecting both consumers and suppliers. This blog explores the critical connection between weather and energy markets, the techniques for accurate weather forecasting, and how these forecasts are integrated into energy trading models.
Energy price volatility can be driven by meteorological factors. For instance, temperature extremes—whether heatwaves in summer or cold spells in winter—can cause sudden spikes in energy demand. During a heatwave, the increased use of air conditioning can lead to higher electricity consumption, while in winter, heating demands surge. These fluctuations in demand often result in price volatility, as energy suppliers scramble to meet the sudden changes in consumption patterns. This can include power plants switching from natural gas to coal for electricity generation. This shift would affect CO2 emissions and overall energy costs.
In addition, adverse weather conditions such as hurricanes, storms, and heavy snowfall can disrupt energy supply chains right across the globe. These disruptions can lead to temporary shortages and drive-up prices. Accurate weather forecasting therefore becomes a critical tool for energy market participants, enabling them to anticipate and mitigate the impacts of such disruptions.
Accurate weather forecasting is crucial for predicting energy market trends and managing price volatility. Several advanced techniques and models can be used to help improve the accuracy of these forecasts:
Numerical Weather Prediction (NWP) models: NWP models use mathematical equations to simulate atmospheric processes. These models are fed with vast amounts of meteorological data, including temperature, humidity, wind speed, and pressure. By running these simulations, meteorologists can predict weather conditions with considerable accuracy.
Statistical models: these models analyse historical weather data to identify patterns and correlations with energy demand and prices. By understanding these historical relationships, statistical models can provide forecasts that help anticipate future market conditions.
Machine Learning and AI: with the advent of big data, machine learning algorithms are increasingly being used to enhance weather forecasting. These algorithms can process vast datasets and identify complex patterns that traditional models might miss. AI-driven models are particularly effective in improving short-term weather forecasts, which are crucial for day-to-day energy market operations.
Ensemble forecasting: this technique involves running multiple simulations with slightly varying initial conditions to account for uncertainties in weather predictions. Ensemble forecasting provides a range of possible outcomes, offering a view of future weather conditions based on probability. This approach helps energy traders and market analysts better manage weather-dependent price volatility risks.
As you can imagine, certain weather events have immediate and deep impacts on energy prices. Here are a few examples:
Heatwaves: during prolonged periods of high temperatures, the demand for air conditioning increases sharply, leading to higher electricity consumption. In regions where power generation is already stretched, this can result in significant price spikes. For example, the UK experienced a heatwave in July 2022, with several days of high temperatures. It was the first time that temperatures exceeded 40c, and electricity prices soared due to unprecedented demand.
Cold Snaps: similar to heatwaves, extreme cold weather also drives up demand - this time, for heating. Natural gas prices, in particular, can see sharp increases during cold snaps as households and businesses ramp up their heating systems. The Texas (USA) freeze in February 2021 is a notable example, where natural gas prices skyrocketed due to surging demand and supply disruptions caused by frozen infrastructure.
Storms and Hurricanes: severe weather events like hurricanes can disrupt energy production and distribution networks. Offshore oil rigs, refineries, and power plants are particularly vulnerable. Hurricane Harvey in 2017, for instance, caused significant disruptions in the Gulf Coast’s energy infrastructure, leading to temporary shortages and price spikes in oil and gas.
Renewable Energy Variability: weather conditions also affect the generation of renewable energy. Solar and wind power generation are highly dependent on sunlight and wind conditions, respectively. Sudden changes in weather can lead to fluctuations in renewable energy output, impacting electricity prices. For example, a cloudy day can reduce solar power generation, leading to increased reliance on other energy sources and potential price increases.
Integrating weather forecasts into energy trading models is essential for managing market risks and capitalising on trading opportunities. Here’s how this integration is achieved:
Real-Time Data Integration: energy traders use real-time weather data to adjust their trading strategies. Advanced software platforms and data analytics tools enable traders to monitor weather forecasts continuously and make informed decisions based on the latest information.
Predictive Analytics: by combining weather forecasts with historical energy market data, predictive analytics models can forecast price movements and demand trends. These models help traders anticipate market shifts and position themselves favourably.
Risk Management: weather-related risks are typical in energy trading. By incorporating weather forecasts into risk management models, traders can identify potential risks and develop mitigation strategies. For instance, they might use hedging techniques to protect against price volatility caused by unexpected weather events.
Scenario Analysis: energy trading models often use scenario analysis to assess the impact of different weather conditions on market dynamics. By simulating various weather scenarios, traders can evaluate potential outcomes and develop contingency plans for extreme weather events.
Analyse energy market impacts with comprehensive weather datasets
There are several case studies that highlight the effective use of weather predictions in navigating energy market shifts:
Texas freeze 2021: during the February 2021 freeze in Texas, energy traders who had integrated weather forecasts into their models were better prepared for the sudden surge in natural gas and electricity prices. Those with accurate short-term weather predictions could capitalise on the price spikes by adjusting their trading positions accordingly.
European heatwave 2019: the summer of 2019 saw record-breaking temperatures across Europe, leading to increased electricity demand for cooling. Traders who had anticipated the heatwave using advanced weather forecasting models profited from the subsequent price increases in electricity markets.
Hurricane Harvey 2017: Hurricane Harvey’s impact on the Gulf Coast’s energy infrastructure was significant. Traders who utilised weather forecasts to predict the hurricane’s path and intensity were able to make strategic decisions, such as diverting supply routes and adjusting trading positions to mitigate losses, and taking advantage of price movements.
Renewable energy forecasting in Germany: Germany’s energy market is heavily reliant on renewable sources, particularly wind and solar. Accurate weather forecasts are crucial for predicting renewable energy output. Traders in the German market use sophisticated models that incorporate weather predictions to optimize their trading strategies and manage the variability of renewable energy generation.
Polar vortex 2014: the polar vortex is a large region of cold, rotating air that encircles Earth’s polar regions. This brought extreme cold to North America, placing a huge strain on natural gas supplies and electricity grids. Prices skyrocketed.
Weather prediction accuracy is now a cornerstone of energy market dynamics, influencing price volatility, demand, and supply. Integrating advanced meteorological data and forecasting models into energy trading is somewhat essential for anticipating market shifts and managing risks. As climate patterns continue to evolve, the ability to accurately predict weather conditions and their impacts on energy markets will become increasingly vital. Stakeholders who leverage these insights will be better equipped to navigate the complexities of the energy market, ensuring stability and profitability in an ever-changing landscape.
Analyse energy market impacts with comprehensive weather datasets
April 24th, 2025
April 23rd, 2025
April 22nd, 2025