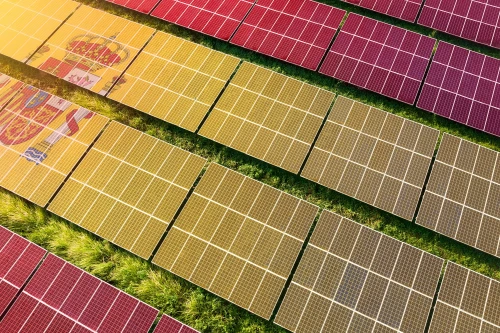
May 9th, 2025
Join our Swedish Energy Day on 20 May – Book now
AI is transforming the energy sector by leveraging weather data to predict and optimise energy production, demand, and pricing.
Weather data is crucial for energy markets because it allows us to examine and forecast production based on the most unpredictable element of renewable energy: the weather. Today, the industry believes that AI is now ready to model and analyse how weather events influence energy generation. If we can use historical data to inform AI about past weather events, AI could analyse and produce assumptions based on how long a weather event may last, predicting when a weather event may end and stop negatively influencing renewable energy production.
We take a look at how AI and machine learning models work with weather data, improving prediction accuracy over traditional forecasting methods.
It’s not just by monitoring how the weather behaves that allows AI to generate best-case and worst-case scenarios based on historical weather data – it may also be able to model demand based on weather scenarios. The Internet of Things (IoT) allows the grid to be connected as one large network, with data being gathered and behavioural patterns examined not only from the source of the power, but also via consumers during periods of good or bad weather. This is useful as AI could be used to analyse and create algorithms based on consumer behaviour, forecasting usage during certain seasons and, therefore, give recommendations to the grid based on this.
This forecasting based on historical weather data can also help address the volatility associated with pricing. Energy trading tends to follow a pattern based on pricing goods in demand or short in demand at a higher price and less in demand at a lower price. Historically, pricing for renewable energy specifically can be quite unpredictable, so using AI to detect fluctuations in the market before they happen based on algorithms could mean pricing has fewer highs and lows because we can predict more accurately the length of a weather event based on previous events and when energy production levels may return to normal.
AI is set to be a crucial technology for renewable energy, not least for those hoping to operate successfully within the energy market frameworks. Below are some specific applications of AI in energy markets:
AI can help to predict the output of a renewable power plant, allowing the grid to become more stable. This is because AI can examine historical output data accurately and make recommendations based on energy storage and when and where energy should be dispatched, making the output more reliable and stable.
The technology can also allow the grid to better balance supply and demand. It does this by examining historical supply and demand mismatches or imbalances and altering renewable energy production to match usage more closely, so the correct amount of energy is generated to fulfil demand.
AI has the potential to examine patterns in energy trading and create accurate predictions based on historical market performance. It could allow energy traders and market operators to make more informed decisions about market elements such as pricing and whether or not to participate in a particular market. This will be particularly advantageous as renewable power stations begin to integrate into the wider smarter grid.
As the global smart grid expands, more and more international collaboration will occur, resulting in knowledge sharing and a greater understanding of renewable energy and its limitations. Stakeholders, policy-makers, and market investors will potentially benefit from AI’s role in weather prediction, as making sense of the data noise will allow them greater decision-making power over potential risk. AI will also be beneficial for stakeholders operating within decentralised grids, which will have a smaller yet more relevant, accurate pool of data to analyse, giving more bespoke projections based on specific historical data and potentially improving operational efficiency.
While AI can be a useful tool in weather forecasting for energy, as a fledgling technology it does have its limitations:
The age-old issue of integrating legacy equipment with new technology is a pressure point that has plagued the energy industry for decades. Technology has, and continues to, develop at a rapid pace, leaving existing equipment with connectivity issues. Traditional plants were never designed to interface with burgeoning new technology. This is particularly prevalent in the smart grid – traditional fuel sources, such as fossil fuel plants, are being integrated with new renewable sources as part of the IoT. This takes us on to accuracy issues, explained below.
The IoT operates by placing tiny sensors onto equipment and monitoring these pieces of technology, feeding back to a central data network where the data is processed and ‘normal’ operating levels determined. When performance differs from ‘normal’, preventative maintenance can be carried out. AI can use these deviating occurrences to identify patterns and predict when failures will occur before they happen. However, sensors can only receive and transmit as much data as it is possible to collect, and legacy equipment is not necessarily set up to allow monitoring for true accuracy. With legacy and modern equipment producing data output that varies wildly across different sources, this can make it difficult for AI to accurately make sense of data coming from these various sources.
These accuracy and integration issues arise from data processing issues. Incomplete or disrupted data gathered from energy plants via the IoT due to legacy equipment can cause data processing to be less accurate than required, meaning the data set AI is working from is not as useful. Therefore, the predictions put forward by AI may not be as robust as if a complete or undisrupted data set is used.
AI holds immense potential for weather data prediction in energy markets, helping to optimise operations, balance supply and demand, and improve decision-making. Despite challenges in integration and data processing, the ongoing advancements in AI and IoT are paving the way for smarter, more resilient energy grids.
Understand the impacts of weather on your power modelling with up to 26 daily updates.
May 9th, 2025
May 8th, 2025
May 7th, 2025